
Extension
In the process of creating AI applications, developers face constantly changing business needs and complex technical challenges. Effectively leveraging extension capabilities can not only enhance the flexibility and functionality of applications but also ensure the security and compliance of enterprise data. Cyberwisdom TalentBot LLMops offers the following two methods of extension:
API-based Extension
Code-based Extension
API-based Extension
Developers can extend module capabilities through the API extension module. Currently supported module extensions include:
-
moderation
-
external_data_tool
Before extending module capabilities, prepare an API and an API Key for authentication, which can also be automatically generated by Cyberwisdom TalentBot LLMops. In addition to developing the corresponding module capabilities, follow the specifications below so that Cyberwisdom TalentBot LLMops can invoke the API correctly.
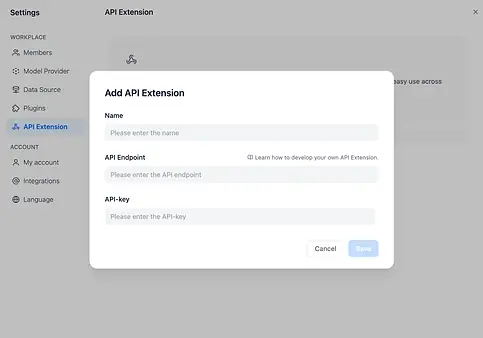
Add API Extension
API Specifications
Cyberwisdom TalentBot LLMops will invoke your API according to the following specifications:
POST {Your-API-Endpoint}
Header
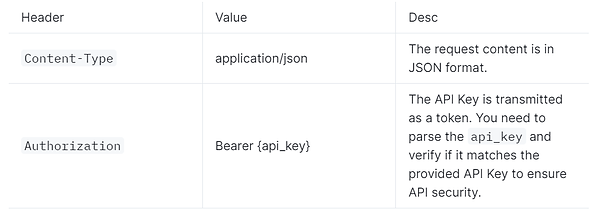
Request Body
{
"point": string, // Extension point, different modules may contain multiple extension points
"params": {
... // Parameters passed to each module's extension point
}
}
API Response
{
... // For the content returned by the API, see the specific module's design specifications for different extension points.
}
Check
When configuring API-based Extension in Cyberwisdom TalentBot LLMops, Cyberwisdom TalentBot LLMops will send a request to the API Endpoint to verify the availability of the API. When the API Endpoint receives point=ping, the API should return result=pong, as follows:
Header
Content-Type: application/json
Authorization: Bearer {api_key}
Request Body
{
"point": "ping"
}
Expected API response
{
"result": "pong"
}
For Example
Here we take the external data tool as an example, where the scenario is to retrieve external weather information based on the region as context.
API Specifications
POST https://fake-domain.com/api/Cyberwisdom TalentBot LLMops/receive
Header
Content-Type: application/json
Authorization: Bearer 123456
Request Body
{
"point": "app.external_data_tool.query",
"params": {
"app_id": "61248ab4-1125-45be-ae32-0ce91334d021",
"tool_variable": "weather_retrieve",
"inputs": {
"location": "London"
},
"query": "How's the weather today?"
}
}
API Response
{
"result": "City: London\nTemperature: 10°C\nRealFeel®: 8°C\nAir Quality: Poor\nWind Direction: ENE\nWind Speed: 8 km/h\nWind Gusts: 14 km/h\nPrecipitation: Light rain"
}
Code demo
The code is based on the Python FastAPI framework.
Install dependencies.
pip install 'fastapi[all]' uvicorn
Write code according to the interface specifications.
from fastapi import FastAPI, Body, HTTPException, Header
from pydantic import BaseModel
app = FastAPI()
class InputData(BaseModel):
point: str
params: dict
@app.post("/api/Cyberwisdom TalentBot LLMops/receive")
async def Cyberwisdom TalentBot LLMops_receive(data: InputData = Body(...), authorization: str = Header(None)):
"""
Receive API query data from Cyberwisdom TalentBot LLMops.
"""
expected_api_key = "123456" # TODO Your API key of this API
auth_scheme, _, api_key = authorization.partition(' ')
if auth_scheme.lower() != "bearer" or api_key != expected_api_key:
raise HTTPException(status_code=401, detail="Unauthorized")
point = data.point
# for debug
print(f"point: {point}")
if point == "ping":
return {
"result": "pong"
}
if point == "app.external_data_tool.query":
return handle_app_external_data_tool_query(params=data.params)
# elif point == "{point name}":
# TODO other point implementation here
raise HTTPException(status_code=400, detail="Not implemented")
def handle_app_external_data_tool_query(params: dict):
app_id = params.get("app_id")
tool_variable = params.get("tool_variable")
inputs = params.get("inputs")
query = params.get("query")
# for debug
print(f"app_id: {app_id}")
print(f"tool_variable: {tool_variable}")
print(f"inputs: {inputs}")
print(f"query: {query}")
# TODO your external data tool query implementation here,
# return must be a dict with key "result", and the value is the query result
if inputs.get("location") == "London":
return {
"result": "City: London\nTemperature: 10°C\nRealFeel®: 8°C\nAir Quality: Poor\nWind Direction: ENE\nWind "
"Speed: 8 km/h\nWind Gusts: 14 km/h\nPrecipitation: Light rain"
}
else:
return {"result": "Unknown city"}
Launch the API service.
The default port is 8000. The complete address of the API is: http://127.0.0.1:8000/api/Cyberwisdom TalentBot LLMops/receivewith the configured API Key '123456'.
uvicorn main:app --reload --host 0.0.0.0
Configure this API in Cyberwisdom TalentBot LLMops.
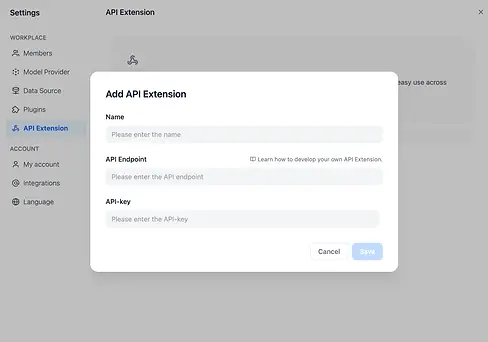
Select this API extension in the App.
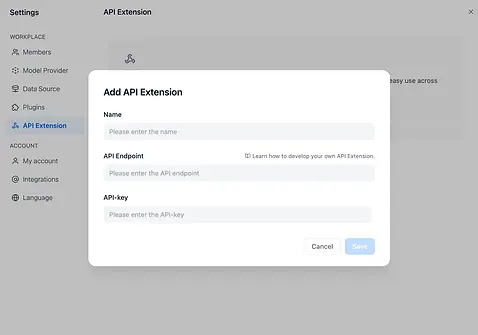
When debugging the App, Cyberwisdom TalentBot LLMops will request the configured API and send the following content (example):
{
"point": "app.external_data_tool.query",
"params": {
"app_id": "61248ab4-1125-45be-ae32-0ce91334d021",
"tool_variable": "weather_retrieve",
"inputs": {
"location": "London"
},
"query": "How's the weather today?"
}
}
API Response:
{
"result": "City: London\nTemperature: 10°C\nRealFeel®: 8°C\nAir Quality: Poor\nWind Direction: ENE\nWind Speed: 8 km/h\nWind Gusts: 14 km/h\nPrecipitation: Light rain"
}
Local debugging
Since Cyberwisdom TalentBot LLMops's cloud version can't access internal network API services, you can use Ngrok to expose your local API service endpoint to the public internet for cloud-based debugging of local code. The steps are:
1.Visit the Ngrok official website at https://ngrok.com, register, and download the Ngrok file.

2.After downloading, go to the download directory. Unzip the package and run the initialization script as instructed:
$ unzip /path/to/ngrok.zip
$ ./ngrok config add-authtoken 你的Token
3.Check the port of your local API service.

Run the following command to start:
$ ./ngrok http [port number]
Upon successful startup, you'll see something like the following:

4.Find the 'Forwarding' address, like the sample domain https://177e-159-223-41-52.ngrok-free.app, and use it as your public domain.
-
For example, to expose your locally running service, replace the example URL http://127.0.0.1:8000/api/Cyberwisdom TalentBot LLMops/receive with https://177e-159-223-41-52.ngrok-free.app/api/Cyberwisdom TalentBot LLMops/receive.
Now, this API endpoint is accessible publicly. You can configure this endpoint in Cyberwisdom TalentBot LLMops for local debugging. For the configuration steps, consult the appropriate documentation or guide.
External_data_tool
When creating AI applications, developers can use API extensions to incorporate additional data from external tools into prompts as supplementary information for LLMs.
Please read API-based Extension to complete the development and integration of basic API service capabilities.
Extension Point
app.external_data_tool.query: Apply external data tools to query extension points.
This extension point takes the application variable content passed in by the end user and the input content (fixed parameters for conversational applications) as parameters to the API. Developers need to implement the query logic for the corresponding tool and return the query results as a string type.
Request Body
{
"point": "app.external_data_tool.query",
"params": {
"app_id": string,
"tool_variable": string,
"inputs": {
"var_1": "value_1",
"var_2": "value_2",
...
},
"query": string | null
}
}
-
Example
{
"point": "app.external_data_tool.query",
"params": {
"app_id": "61248ab4-1125-45be-ae32-0ce91334d021",
"tool_variable": "weather_retrieve",
"inputs": {
"location": "London"
},
"query": "How's the weather today?"
}
}
API Response
{
"result": string
}
-
Example
{
"result": "City: London\nTemperature: 10°C\nRealFeel®: 8°C\nAir Quality: Poor\nWind Direction: ENE\nWind Speed: 8 km/h\nWind Gusts: 14 km/h\nPrecipitation: Light rain"
}
Moderation Extension
This module is used to review the content input by end-users and the output from LLMs within the application, divided into two types of extension points.
Please read API-based Extension to complete the development and integration of basic API service capabilities.
Extension Point
app.moderation.input: End-user input content review extension point. It is used to review the content of variables passed in by end-users and the input content of dialogues in conversational applications.
app.moderation.output: LLM output content review extension point. It is used to review the content output by LLM. When the LLM output is streaming, the content will be requested by the API in chunks of 100 characters to avoid delays in review when the output content is lengthy.
app.moderation.input
Request Body
{
"point": "app.moderation.input",
"app_id": string,
"inputs": {
"var_1": "value_1",
"var_2": "value_2",
...
},
"query": string | null
}
}
-
Example
{
"point": "app.moderation.input",
"params": {
"app_id": "61248ab4-1125-45be-ae32-0ce91334d021",
"inputs": {
"var_1": "I will kill you.",
"var_2": "I will fuck you."
},
"query": "Happy everydays."
}
}
API Response
{
"flagged": bool,
"action": string,
"preset_response": string,
"inputs": {
"var_1": "value_1",
"var_2": "value_2",
...
},
"query": string | null
}
-
Example
action=direct_output
{
"flagged": true,
"action": "direct_output",
"preset_response": "Your content violates our usage policy."
}
action=overrided
{
"flagged": true,
"action": "overrided",
"inputs": {
"var_1": "I will *** you.",
"var_2": "I will *** you."
},
"query": "Happy everydays."
}
app.moderation.output
Request Body
{
"point": "app.moderation.output",
"params": {
"app_id": string,
"text": string
}
}
-
Example
{
"point": "app.moderation.output",
"params": {
"app_id": "61248ab4-1125-45be-ae32-0ce91334d021",
"text": "I will kill you."
}
}
API Response
{
"flagged": bool,
"action": string,
"preset_response": string,
"text": string
-
Example
action=direct_output
{
"flagged": true,
"action": "direct_output",
"preset_response": "Your content violates our usage policy."
}
action=overrided
{
"flagged": true,
"action": "overrided",
"text": "I will *** you."
}
Code-based Extension
For developers deploying Cyberwisdom TalentBot LLMops locally who wish to implement extension capabilities, there is no need to rewrite an API service. Instead, they can use Code-based Extension, which allows for the expansion or enhancement of the program's capabilities in the form of code (i.e., plugin capabilities) on top of Cyberwisdom TalentBot LLMops's existing features, without disrupting the original code logic of Cyberwisdom TalentBot LLMops. It follows certain interfaces or specifications to ensure compatibility and pluggability with the main program. Currently, Cyberwisdom TalentBot LLMops has opened up two types of Code-based Extensions, which are:
-
Add a new type of external data tool
-
Expand sensitive content review policies
On the basis of the above functions, you can follow the specifications of the code-level interface to achieve the purpose of horizontal expansion.